Artificial neural network and Deep learning are related fields, but they are not exactly the same thing. Artificial neural networks are a type of machine learning model that uses a layered approach to simulate the behavior of neurons in the brain. Deep learning is a subset of machine learning that specifically uses deep neural networks with many layers to perform complex tasks.
Here are some blog posts that explore the differences and advancements of deep learning and artificial neural networks:
Overview:
Artificial neural network and deep learningare two related fields within machine learning. Artificial neural networks use a layered approach to simulate the behavior of neurons in the brain, while deep learning uses deep neural networks with many layers to perform complex tasks. Deep learning has seen recent advancements that have been transformative and have the potential to transform various industries. It is important to keep up with the latest advancements and trends in these fields to stay on top of the rapidly evolving field of machine learning.
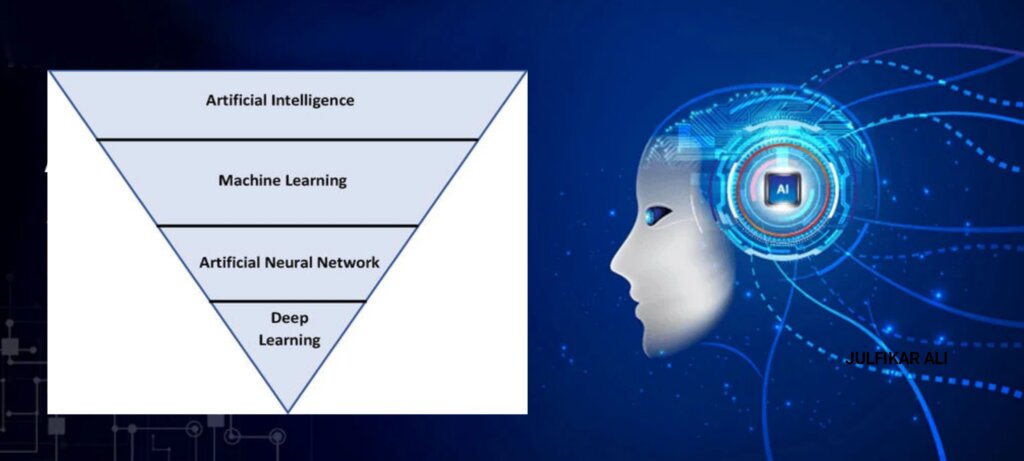
Advancement of artificial neural network and deep learning
- “Deep Learning vs Neural Networks: What’s the Difference?” by Adrian Rosebrock on PyImageSearch. This post provides a clear and concise explanation of the differences between deep learning and neural networks, as well as their respective strengths and weaknesses.
- “Why Deep Learning Is Suddenly Changing Your Life” by Fortune. This article discusses the recent advancements in deep learning and how they are transforming industries such as healthcare, finance, and transportation.
- “The State of Deep Learning in 2021” by Christof Henkel on Towards Data Science. This post provides an overview of the latest advancements and trends in deep learning, including the use of transformers, GANs, and reinforcement learning.
- “Artificial Neural Networks vs. Deep Learning Networks: What’s the Difference?” by Nikhil Kumar on Analytics Insight. This post explores the differences between artificial neural networks and deep learning networks, including their architectures and applications.
- “Deep Learning vs Machine Learning vs Artificial Intelligence” by Ritwik Gupta on Analytics Vidhya. This post provides a comprehensive overview of the differences between deep learning, machine learning, and artificial intelligence, as well as their applications and limitations.
- “Deep Learning: The Good, the Bad, and the Ugly” by James Le on Medium. This post explores the pros and cons of deep learning, including its ability to learn complex patterns and its potential for overfitting and lack of interpretability.
- “Neural Networks vs. Deep Learning: What’s the Difference?” by Aileen Nielsen on IBM Developer. This post provides a detailed explanation of the differences between neural networks and deep learning, including their architectures, training methods, and applications.
- “A Brief History of Neural Networks and Deep Learning” by Jason Brownlee on Machine Learning Mastery. This post provides a historical overview of neural networks and deep learning, including their origins and key milestones in their development.
- “The Difference Between Machine Learning and Deep Learning” by Daniel Seita on Data Science 101. This post provides a clear explanation of the differences between machine learning and deep learning, including their architectures, data requirements, and applications.
- “Why Deep Learning is the Future of AI” by Martin Görner on Google Cloud Blog. This post explores the reasons why deep learning is likely to become the dominant approach to AI in the future, including its ability to handle large datasets and its potential for creating new breakthroughs in AI research.
Conclusion
In conclusion, deep learning and artificial neural networks are related fields within machine learning, but deep learning specifically refers to the use of deep neural networks with many layers. Both fields have their own strengths and weaknesses, applications and limitations. However, the recent advancements in deep learning have been particularly transformative, as they have enabled the development of new breakthroughs in AI research and have the potential to transform various industries. Overall, keeping up with the latest advancements and trends in deep learning and artificial neural networks is crucial for staying on top of the rapidly evolving field of machine learning. for more…
FAQ
- What is the importance neural network in the advancement of technology
Neural networks are a type of machine learning algorithm that have played a critical role in advancing various fields of technology, such as computer vision, natural language processing, speech recognition, and robotics. Their ability to recognize patterns and make predictions based on large datasets has made them valuable tools in areas such as image recognition, fraud detection, recommendation systems, and many other applications. Their advancement has led to significant improvements in technological fields, allowing machines to perform complex tasks that were once impossible, and opening up new possibilities for innovation and progress.
- Is deep learning most advanced from AI?
Deep learning is a subset of artificial intelligence (AI) and has been one of the most significant advances in the field in recent years. It uses neural networks with multiple layers to analyze and learn from complex data sets, such as images, sounds, and texts. While deep learning has achieved remarkable success in areas such as computer vision, speech recognition, and natural language processing, it is not necessarily the most advanced form of AI. There are still many challenges to be addressed, and researchers are continuously exploring new techniques and approaches to improve AI systems’ performance and capabilities.
- What is the most advanced neural network?
It is difficult to say which is the most advanced neural network as researchers are continually developing new and improved architectures to tackle complex problems. Some of the most advanced neural networks to date include Transformer networks, which have revolutionized natural language processing tasks, GPT-3, a language model with over 175 billion parameters that can perform various language tasks, and AlphaGo, a neural network that defeated the world champion at the game of Go, showcasing the power of deep reinforcement learning. However, as research in the field continues, new and even more advanced neural networks are likely to be developed.
Differences between AI, Deep Learning vs Machine Learning, and Artificial Neural Networks ?
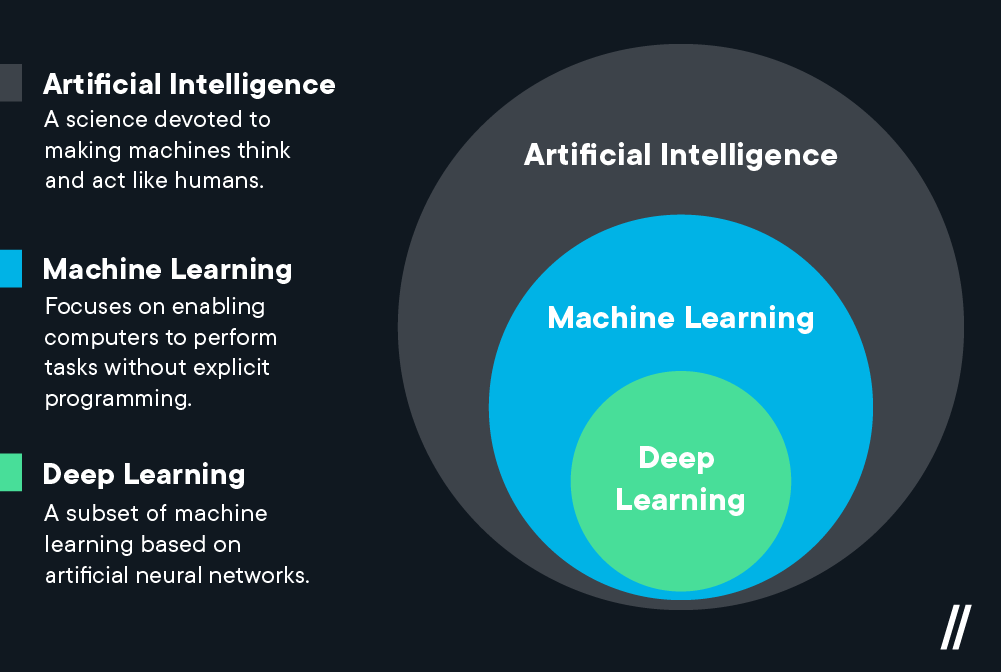